Detecting credit card fraud is a huge challenge for businesses, but it is crucial to protect your customers and your bottom line. In this blog post, we will discuss how machine learning can be used to detect fraudulent transactions. We will also provide examples of how this technology is being used by businesses today.
How to use machine learning for credit card fraud detection
Businesses today are turning to machine learning algorithms to detect fraudulent credit card transactions. By training a machine learning model on historical data, businesses can develop a system that can flag potentially fraudulent transactions in real-time. This is an important step in protecting your customers and preventing losses.
There are many different ways to approach this problem, but one popular method is to use a neural network. Neural networks are well-suited for this task because they can learn complex patterns in data. In addition, neural networks are capable of handling a large amount of data, which is important when dealing with credit card transactions.
There are many different types of neural networks, but one that is particularly well-suited for this task is the long short-term memory (LSTM) network. LSTM networks are a type of recurrent neural network (RNN). RNNs are well-suited for sequential data, such as credit card transactions. This is because they can remember information from previous transactions when making predictions about future transactions.
LSTM networks have been shown to be very effective at detecting credit card fraud. In one study, an LSTM network was able to achieve a 99.97% accuracy rate in detecting fraudulent transactions. This is an impressive result, and it shows that machine learning can be used to effectively detect credit card fraud.
How to use Python for credit card fraud detection?
Python is a popular programming language that is well-suited for data science and machine learning. It has many different libraries that can be used for this task.
One popular library for machine learning is TensorFlow. TensorFlow is a library that was developed by Google. It is used for machine learning and deep learning. TensorFlow can be used to train neural networks for credit card fraud detection.
Another popular library for machine learning is scikit-learn. Scikit-learn is a library that provides a variety of machine learning algorithms. We can use scikit-learn to train a logistic regression model for credit card fraud detection.
Both TensorFlow and scikit-learn are open-source libraries that can be used for free.
How data science can help with credit card fraud detection
Data science is a powerful tool that can be used to detect credit card fraud. Data science involves using techniques such as data mining, machine learning, and statistics. By using these techniques, businesses can develop systems that can flag potentially fraudulent transactions.
What is logistic regression and how to use it to detect credit card fraud?
Logistic regression is a type of machine learning algorithm that can be used for classification tasks. Logistic regression works by training a model on historical data. The model learns to identify patterns in the data that are associated with fraud. Once the model is trained, it can be used to predict whether a new transaction is likely to be fraudulent.
To train a logistic regression model, we need to provide a dataset that contains historical data about credit card transactions. The dataset should contain information about the transaction, such as the amount, the date, and the location. It should also contain information about whether the transaction was fraudulent.
Once the model is trained, we can use it to predict whether new transactions are likely to be fraudulent. To do this, we need to provide the model with information about the new transaction. The model will then use its learned patterns to predict whether the transaction is likely to be fraudulent.
If the model predicts that the transaction is likely to be fraud, then we can take steps to prevent the fraud from occurring. For example, we could contact the customer to confirm the transaction.
Closing thoughts
Credit card fraud is a huge challenge for businesses, but machine learning can be used to effectively detect it. This is an important step in protecting your customers and preventing losses. Python is a popular programming language that is well-suited for data science and machine learning. It has many different libraries that can be used for this task.
Further questions
What's your question? Ask it in the discussion forum
Have an answer to the questions below? Post it here or in the forum

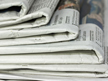
"It Ends with Us" star Blake Lively filed a legal complaint against her costar, Justin Baldoni, for sexual harassment and for conspiring to damage her reputation.
She taught them how stocks and savings bonds work and encouraged them to be self-reliant, skills that have help them reach their own milestones.
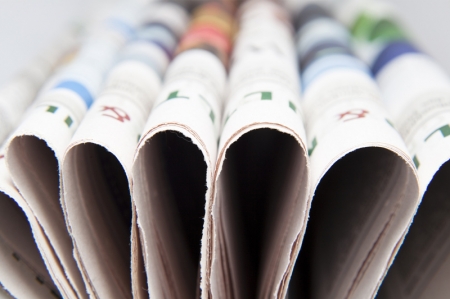