If you are looking for a comprehensive guide to learning Python for quantitative finance, look no further! In this blog post, we will discuss everything you need to know in order to get started. We will cover topics such as installing Python, working with financial data, using libraries for quantitative finance, and more. So whether you are a beginner or an experienced quant, this blog post is for you.
What is Python?
Python is a programming language that has gained popularity in recent years for its versatility and ease of use. For finance, Python can be used for data analysis, algorithmic trading, and even building financial models. In this blog post, we will focus on the latter two applications.
Installing Python is relatively straightforward and there are a number of tutorials available online. For this blog post, we will be using the Anaconda distribution of Python which can be downloaded here. Anaconda comes with a number of useful libraries for data science and quantitative finance so it is a good choice for our purposes.
Once you have installed Anaconda, you should open up the Anaconda Navigator application and launch the Jupyter Notebook. The Jupyter Notebook is a web-based interactive development environment for Python where we can write and execute code cells. We will be using the Jupyter Notebook throughout this blog post so it is important to have it up and running.
How to get started with working with financial data in Python
A lot of the time, the first step in any quantitative finance project is to obtain data. This data can be from a variety of sources such as financial statements, stock price data, economic data, etc. For this blog post, we will be using stock price data from Yahoo Finance.
There are a number of ways to get Yahoo Finance data into Python. The easiest way is to use the yfinance library which can be installed using the following command:
pip install yfinance
“`
Once you have installed the yfinance library, you can use it to fetch data for a given ticker symbol. For example, if we wanted to get Apple’s stock price data, we could use the following code:
“`python
import yfinance as yf
data = yf.download(“AAPL”, start=”2020-01-01″, end=”2020-02-01″)
“`
In the code above, we first import the yfinance library and then use the download function to fetch data for the AAPL ticker from Yahoo Finance. The start and end arguments specify the dates that we want to get data for. The data variable contains a pandas DataFrame with the stock price data.
We can also use the yfinance library to get other types of data such as economic data.
Libraries for quantitative finance
In addition to the yfinance library, there are a number of other libraries that can be useful for quantitative finance. Some of these libraries include:
* pandas-datareader: Used for fetching data from a variety of sources including Yahoo Finance, Google Finance, and more.
* numpy: Used for numerical computing in Python.
* scipy: Used for scientific computing in Python.
* matplotlib: Used for plotting and visualizing data in Python.
* seaborn: Used for statistical data visualization.
* statsmodels: Used for statistical modeling.
*scikit-learn: Used for machine learning in Python.
These are just some of the most popular libraries used in quantitative finance. There are many other libraries available and we encourage you to explore them on your own.
Conclusion
In conclusion, Python is a powerful programming language that can be used for a variety of purposes in quantitative finance. We hope this blog post has given you a good introduction to working with financial data in Python.
Further questions
What's your question? Ask it in the discussion forum
Have an answer to the questions below? Post it here or in the forum
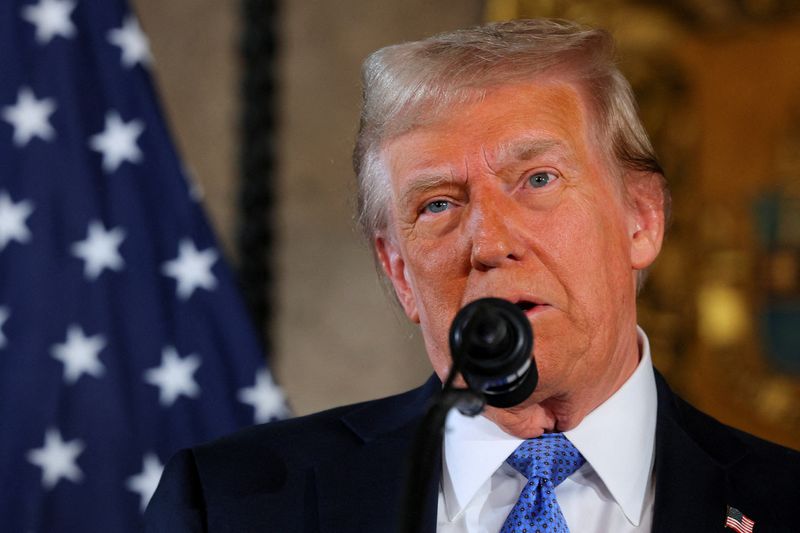
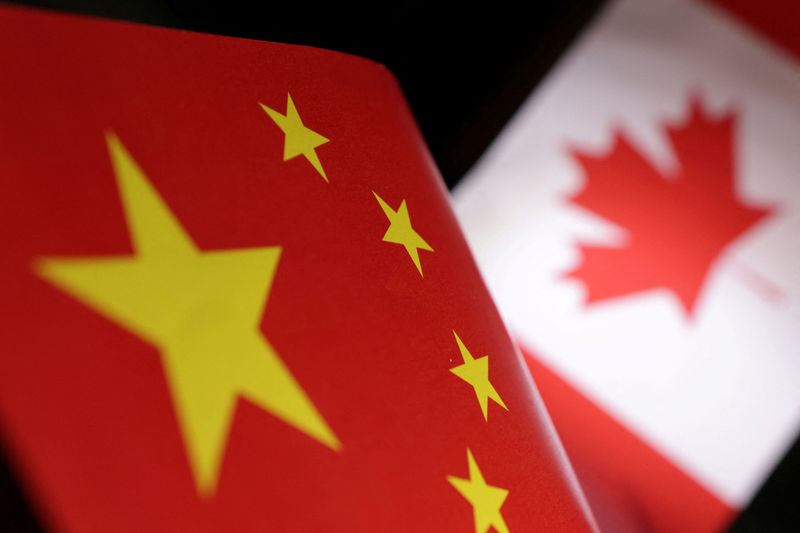
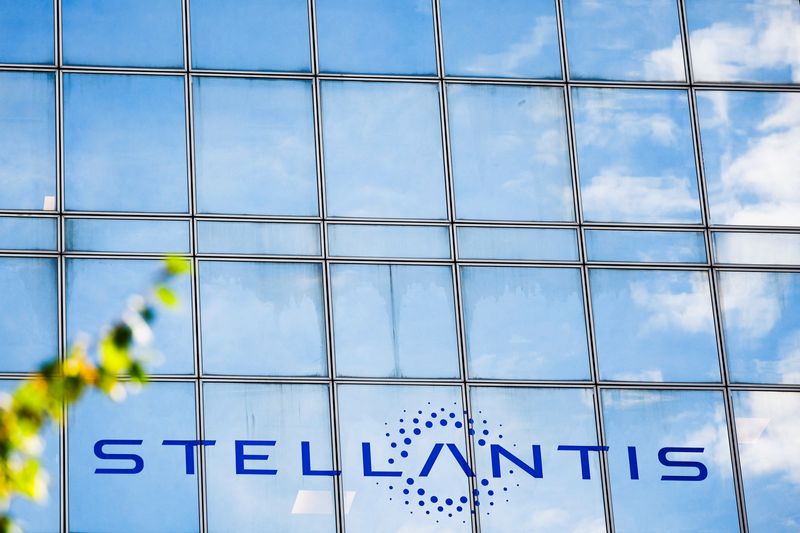
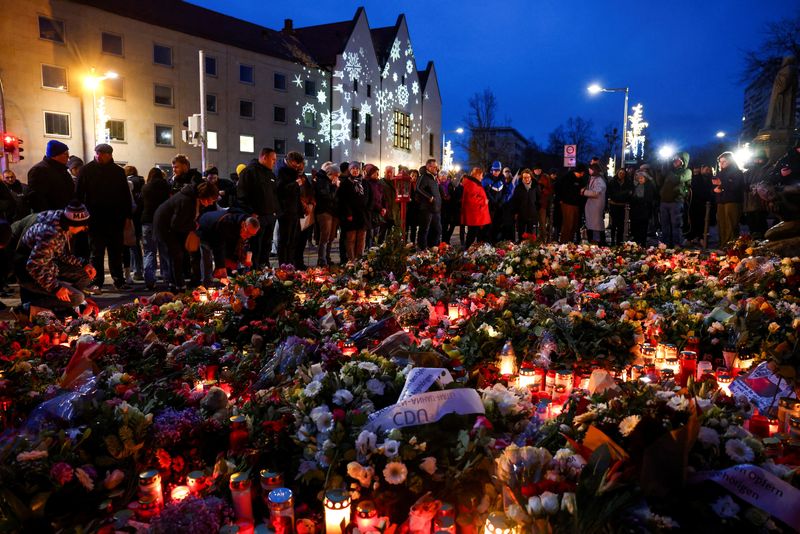
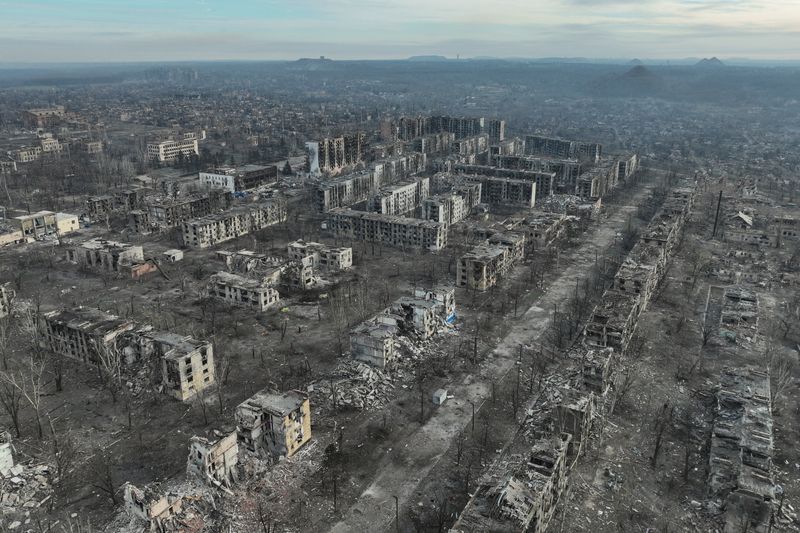