Data science and machine learning have allowed analysts to process data at more efficient rates. Due to the abundance of tools at disposal currently, the process has become much straightforward. Data science has contributed to many other fields, including economics. More particularly, it has enhanced how analysts use econometrics to process a large amount of data.
However, econometrics and data science are different. Before understanding that, however, it is crucial to look at what both of these are.
What is Econometrics?
The term econometrics refers to the quantitative application of mathematical and statistical models in the field of economics. It explores the use of those methods to test and analyze economic data. Econometrics can be crucial in examining and developing economic theories or models. On top of that, it can also help analysts with applying existing economic models to their research.
The field of econometrics in economics has existed for a long time. The term itself comes from the work of Ragnar Frisch, who is a founding father of the Econometric Society, along with Lawrence Klein and Simon Kuznets. Their work in econometrics also earned them a Nobel Prize in economics in 1971. There are several subdivisions and specializations within econometrics, which have come into existence over time.
Econometrics involves the use of statistical tools to test various economic theories and hypotheses. These tools may include regression analysis, correlation analysis, probability, statistical inference, etc. Analysts must obtain and analyze a set of data for all these tools. Therefore, the application of data science can be crucial to econometrics.
What is Data Science?
In the simplest terms, data science entails the use of science to analyze and process data. However, it is more complicated. Data science is the field of study that involves applying algorithms, machine learning principles, etc., to study raw data. Based on the users’ needs, it can identify and analyze a large amount of data and provide processed information.
The increase in the amount of data available has been a crucial factor in the need for data science. It can be beneficial in many fields, for example, predictive analysis, prescriptive analysis, pattern analysis, etc. Through AI, machine learning, deep learning, or similar tools, the process becomes much simpler and time-saving.
Data science also deals with many fields of science. It involves using mathematics and statistics, along with computer and information science. Due to the similar areas of focus, data science and econometrics also connect in different ways. However, both of them are fundamentally different from each other due to several reasons.
What is the difference between Econometrics and Data Science?
As mentioned, both econometrics and data science involve using statistics and mathematics. Due to these, both of the fields have some similarities. However, they also differ from each other in various critical regards. Econometrics involves the use of statistical and mathematical tools in economics, business, and finance. The field studies the relationship between variables and emphasizes predictive or correlative analysis.
On the other hand, data science also involves using mathematics and statistics. However, it also includes other fields, most importantly computer and information science. Data science usually focuses on high speed and accuracy. Furthermore, it emphasizes processing information and identifying patterns. Overall, it deals with how information is processed.
Conclusion
Econometrics is the field of economics that applies mathematical and statistical tools to economic analysis. It deals with using several tools to test or develop economic theories. Data science involves the use of computers and information science to process data. Usually, this data is of a mathematical or statistical nature. Both fields have some commonalities but also differ from each other.
Further questions
What's your question? Ask it in the discussion forum
Have an answer to the questions below? Post it here or in the forum
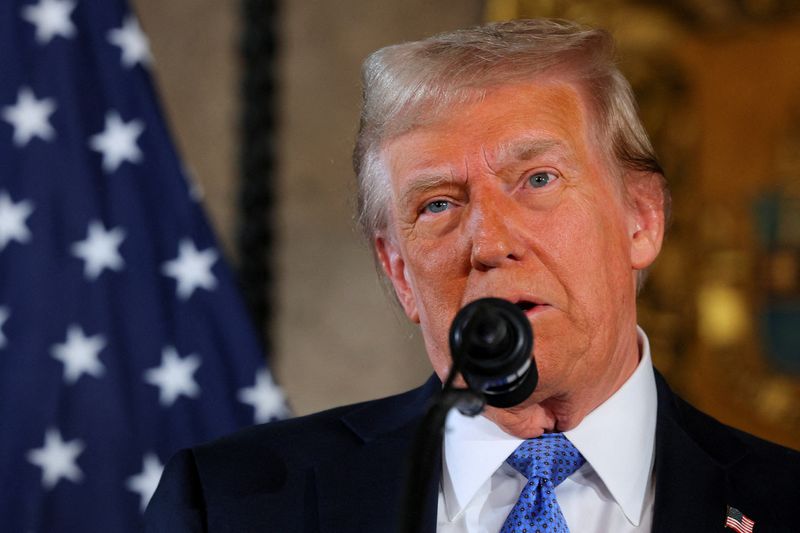
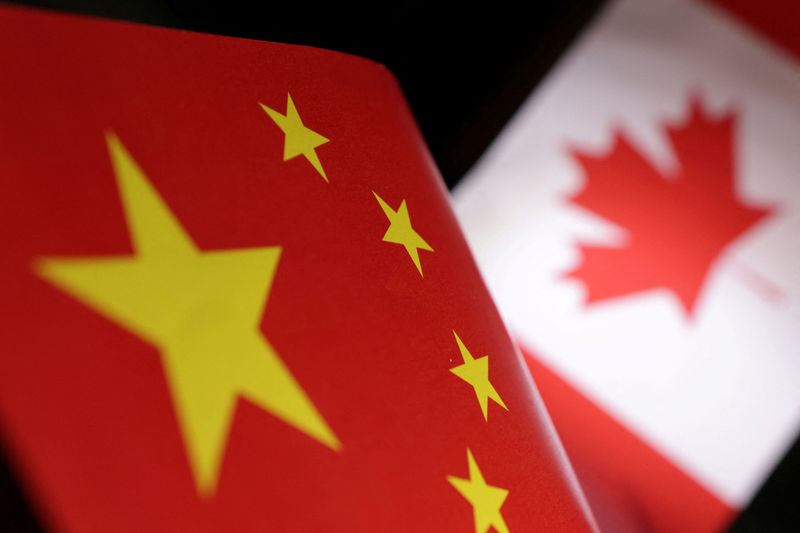
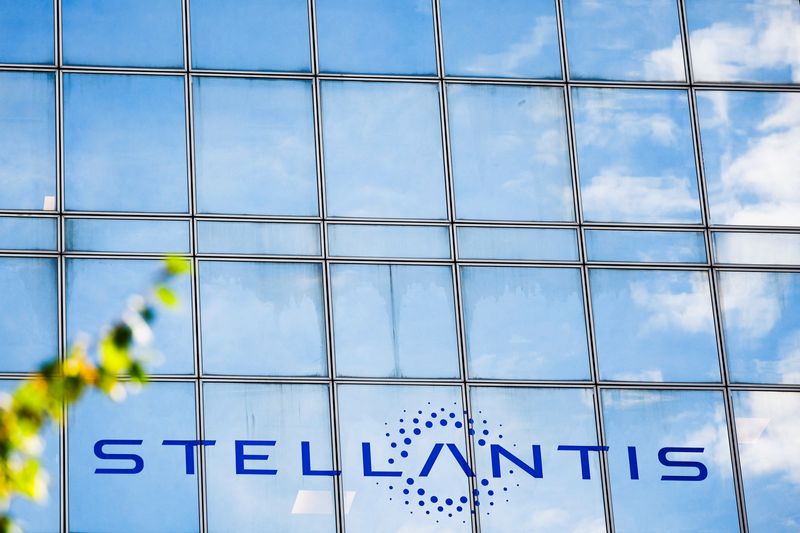
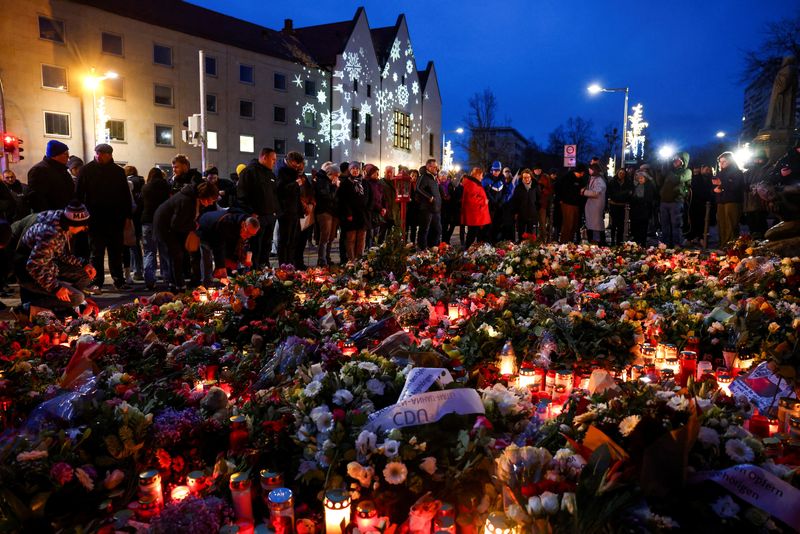
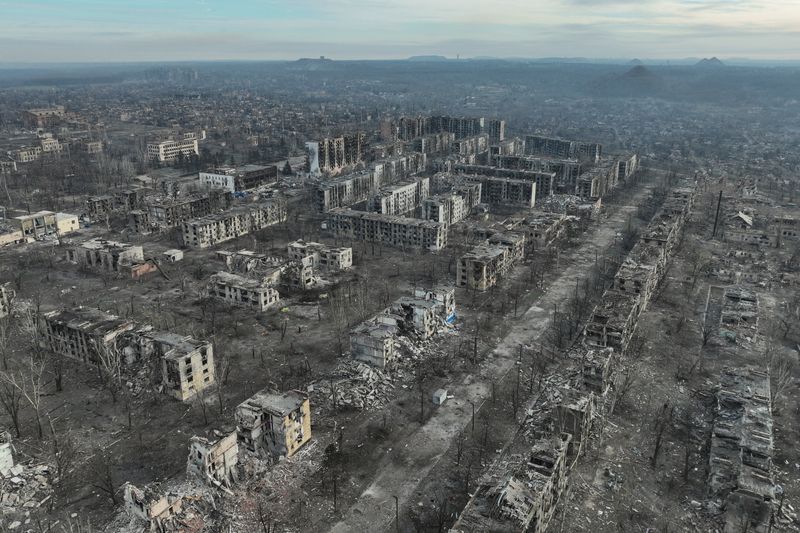