A trading system is a set of rules that a trader uses to make buy and sell decisions. It’s commonly believed that a profitable trading system should be simple, with few parameters, so that it can be easily understood and followed. It is also important for the system to be robust, meaning that it performs well in different market conditions. Reference [1], however, demonstrated that, contrary to conventional wisdom, a complex trading system can offer superior risk-adjusted returns. The authors achieved that by using Machine Learning techniques.
In this article, we offer some new theoretical insight into the expected out-of-sample behavior of machine learning portfolios. Building on recent advances in the theory of high complexity models from the machine learning literature, we demonstrate a theoretical “virtue of complexity” for investment strategies derived from machine learning models. Contrary to conventional wisdom, we prove that market timing strategies based on ridgeless least squares generate positive Sharpe ratio improvements for arbitrarily high levels of model complexity. In other words, the performance of machine learning portfolios can be theoretically improved by pushing model parameterization far beyond the number of training observations, even when minimal regularization is applied. We provide a rigorous foundation for this behavior rooted in techniques from random matrix theory. We complement these technical developments with intuitive descriptions of the key statistical mechanisms at play.
So should a trading system be simple and intuitive or complex?
We believe that whichever approach is taken, it is important that the system has been tested over time and is consistently profitable before risking any real capital.
The article also highlighted an important point that is not discussed very often in the academic literature,
We demonstrate that out-of-sample R2 from a prediction model is generally a poor measure of its economic value. We prove that a market timing model can earn large economic profits when R2 is large and negative. This naturally recommends that the finance profession focus less on evaluating models in terms of forecast accuracy and more on evaluating in economic terms; for example, based on Sharpe ratio of the associated strategy.
In short, an analytical technique should be evaluated based on the strategy performance and not on its predictive power.
References
[1] Kelly, Bryan T. and Malamud, Semyon and Zhou, Kangying, The Virtue of Complexity in Machine Learning Portfolios (2021). Swiss Finance Institute Research Paper No. 21-90, https://ssrn.com/abstract=3984925
Further questions
What's your question? Ask it in the discussion forum
Have an answer to the questions below? Post it here or in the forum
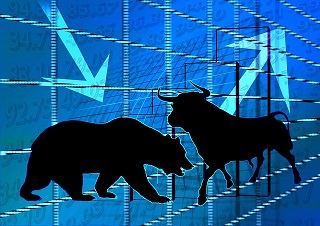
VANCOUVER, British Columbia, Nov. 21, 2024 (GLOBE NEWSWIRE) — Central 1 Credit Union (Central 1) today reported third quarter performance reflecting steady financial results across business lines, consistent with plans and expectations. “Our stable third quarter results were in line with our expectations,” said Sheila…

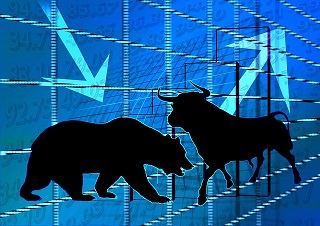
HAMILTON, Bermuda — Aspen Insurance Holdings Limited (“Aspen” or the “Company”) has priced an underwritten public offering of 8,000,000 Depositary Shares (the “Depositary Shares”), each of which represents a 1/1,000th interest in a share of the Company’s newly designated 7.00% Perpetual Non-Cumulative Preference Shares (the…
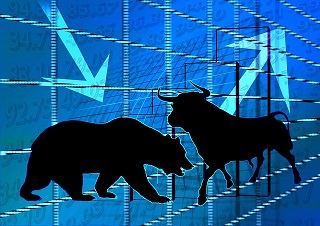
TORONTO — Postmedia Network Canada Corp. (“Postmedia” or the “Company”) today released financial information for the three months and year ended August 31, 2024. “While we continue to operate in a challenging advertising marketplace dominated by large, foreign media platforms, Postmedia achieved some important milestones…
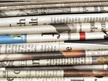