Follow us on LinkedIn
In-sample overfitting is a serious problem when designing trading strategies. This is because a strategy that worked well in the past may not work in the future. In other words, the strategy may be too specific to the conditions that existed in the past and may not be able to adapt to changing market conditions.
One way to avoid in-sample overfitting is to use out-of-sample testing. This is where you test your strategy on data that was not used to develop the strategy. This will give you a more accurate idea of how the strategy will perform in the future. Another way to avoid in-sample overfitting is to use cross-validation techniques. This is where you split your data into multiple sets and train and test your strategy on each set. This will give you a more robust measure of how your strategy will perform.
Reference [1] examined how well the in-sample optimized trading strategies perform out of sample,
This paper analyzed the impact of hyperparameter settings on the predictive performance of support vector machines for stock price movement directions. We tested different settings of hyperparameters in an extensive grid search, applying 10-fold cross-validation for the training set and evaluating the classification performance and strategies’ profitability for out-of-sample data.
The paper concluded that out-of-sample accuracy decreased significantly,
The results indicated that most models had a high in-sample accuracy but only around 50% when applied to out-of-sample data. Nonetheless, a significant proportion of the models managed to outperform the buy-and-hold strategy in terms of profitability. Specifically, a number of models had an out-of-sample F1 Score close to 65%, however the models that yielded the best profitabilities followed a chaotic behavior, as the out-of-sample performance of the models showed significant oscillations for small variations on the hyperparameters.
It’s noteworthy that the most profitable strategies are sensitive to system parameters. This is a cause for concern.
Let us know what you think in the comments below.
References
[1] Yaohao Penga, Joao Gabriel de Moraes Souza, Chaos, overfitting and equilibrium: To what extent can machine learning beat the financial market? Preprint submitted to XXII Brazilian Finance Meeting
Further questions
What's your question? Ask it in the discussion forum
Have an answer to the questions below? Post it here or in the forum
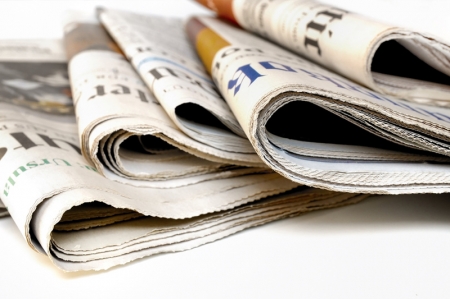
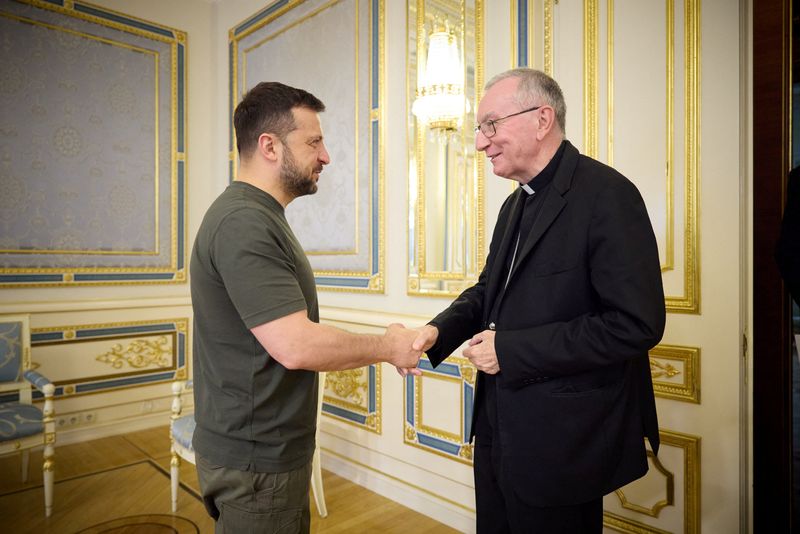
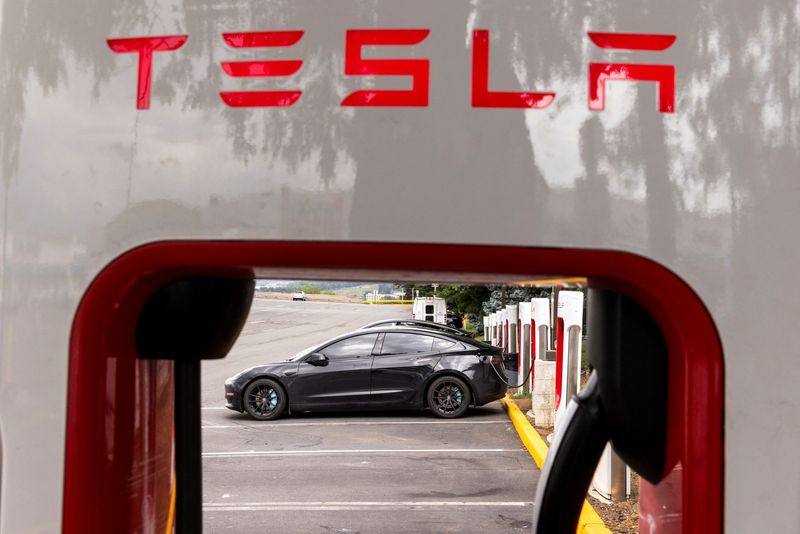
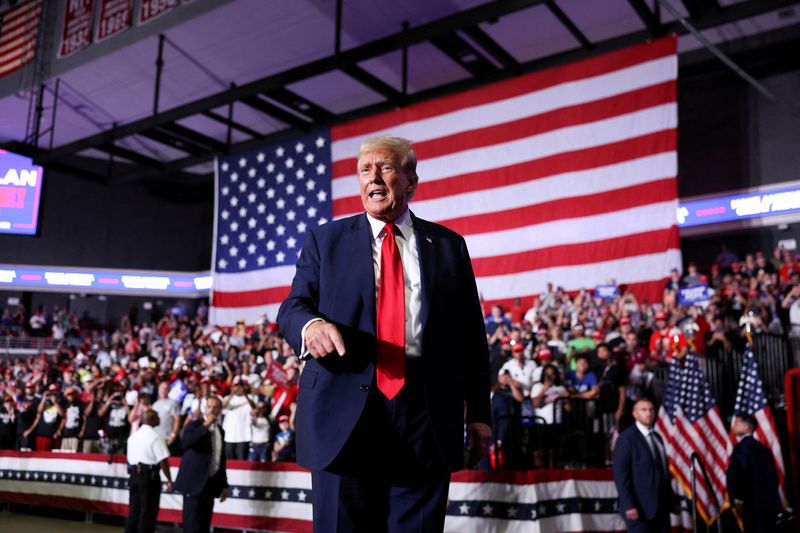
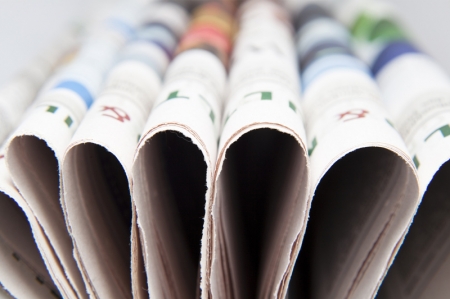