Data science and machine learning are advancing at a rapid pace. They’re now being applied in areas as diverse as healthcare, retail, marketing, and finance. However, a key question that still needs to be answered is: how much data do you need to train these models?
The answer, it turns out, is not always more data. In some cases, using too much data can actually hurt the performance of your machine learning models. In this context, Reference [1] argued that more data is not always better,
Managers often believe that collecting more data will continually improve the accuracy of their machine learning models. However, we argue in this paper that when data lose relevance over time, it may be optimal to collect a limited amount of recent data instead of keeping around an infinite supply of older (less relevant) data. In addition, we argue that increasing the stock of data by including older datasets may, in fact, damage the model’s accuracy. Expectedly, the model’s accuracy improves by increasing the flow of data (defined as data collection rate); however, it requires other tradeoffs in terms of refreshing or retraining machine learning models more frequently.
The paper also pointed out that the value of a firm does not scale with its stock of data,
This result, coupled with the fact that older datasets may deteriorate models’ accuracy, suggests that created business value doesn’t scale with the stock of available data unless the firm offloads less relevant data from its data repository. Consequently, a firm’s growth policy should incorporate a balance between the stock of historical data and the flow of new data.
What implication does this paper have for trading and portfolio management? Should we use more data?
The short answer is probably no. In fact, using more data can actually lead to sub-optimal results. The reason is that, in the financial world, data is often noisy and contains a lot of irrelevant information. If you use too much data, your machine learning models will end up picking up on this noise, which can lead to sub-optimal results.
So how do we use data for trading? Let us know in the comments below.
References
[1] Valavi, Ehsan, Joel Hestness, Newsha Ardalani, and Marco Iansiti. Time and the Value of Data. Harvard Business School Working Paper, No. 21-016, August 2020. (Revised November 2021.)
Further questions
What's your question? Ask it in the discussion forum
Have an answer to the questions below? Post it here or in the forum
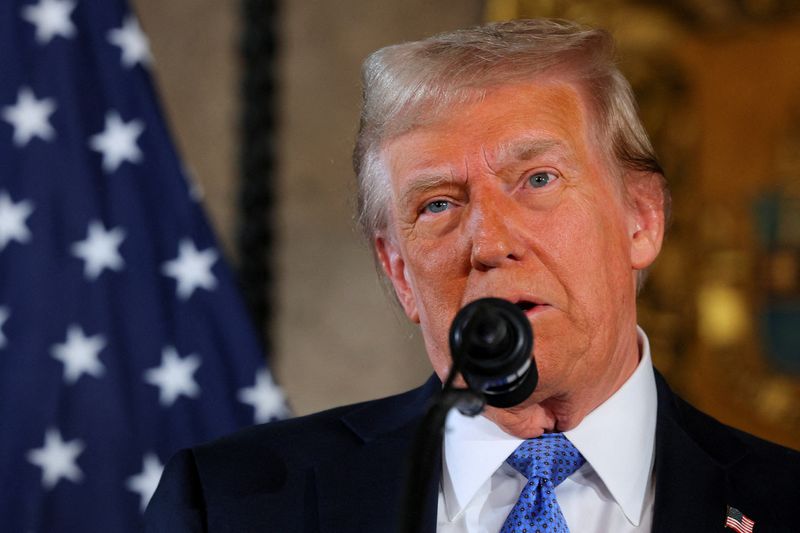
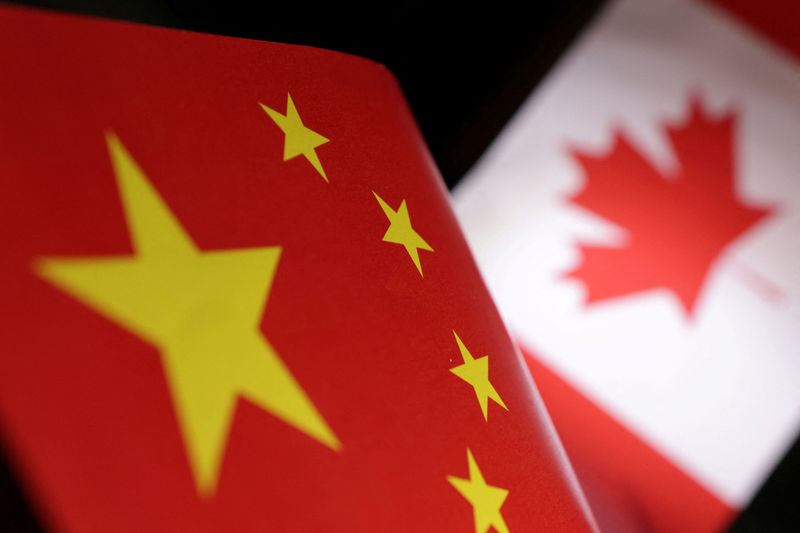
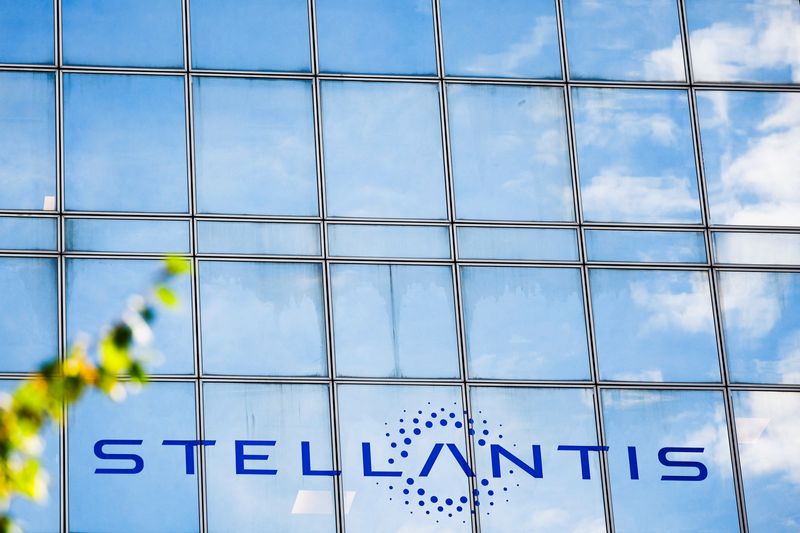
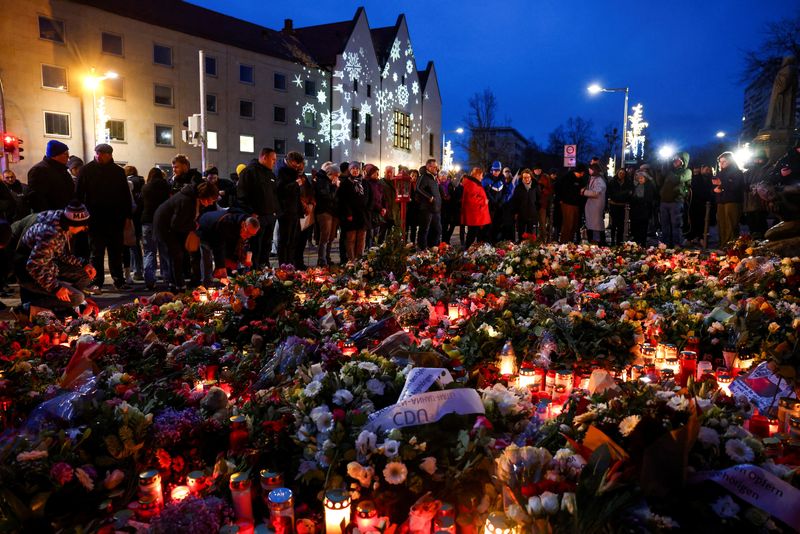
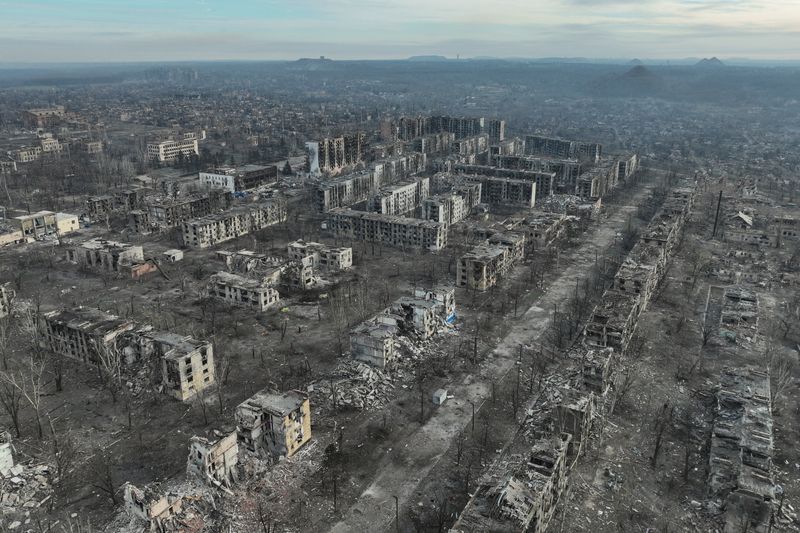