Follow us on LinkedIn
The unpredictability of the markets is a well-known fact. Despite this, many traders and portfolio managers continue to try to predict market volatility and manage their risks accordingly. Usually, econometric models such as GARCH are used to forecast market volatility.
In recent years, machine learning has been shown to be capable of predicting market volatility with accuracy. Reference [1] explored how machine learning can be used in this context. The findings are,
First, we find that a limited number of predictors, namely, the current month realized volatility, idiosyncratic volatility, bid-ask spread and returns, respectively, account for most of the predictive power of the models. Indeed, these are the same variables that are empirically found in other studies to be most important for predicting expected returns confirming the risk-return trade-off in finance.
Second, we show that machine learning methods not only properly can capture the order and magnitude of the impact for each predictor but also the direction of these impacts, as well as the interactions between predictors without any pre-specified interaction effects in the models. In particular, our results indicate that machine learning methods can account for the stylized facts about volatility. We find that large values of the current period volatility have large and positive impact on the next period volatility, whereas, small values of the current period volatility have small and negative impact on the next period volatility…
Third, we show that an LSTM model, which captures the temporal dependence of predictors, can outperform the feedforward neural network and regression tree, which rely on the most recent information in the predictors.6 In particular, our LSTM model with only volatility and return as predictors but up to one year into the past, performs as good as an LSTM model with the full set of predictors and the same number of lags. One can think of our LSTM model as an alternative to the GARCH type models except that we do not need to impose any distributional assumption. However, similar to the GARCH type models we need to find the optimum number of lags through training different models across a range of lags.
In short, the aim of the paper was to demonstrate the potential of machine learning for modeling market volatility. In particular, the authors have shown how the LSTM model can be used to predict market volatility and manage risks. The results suggest that this is a promising alternative approach to traditional econometric models like GARCH.
References
[1] Filipovic, Damir and Khalilzadeh, Amir, Machine Learning for Predicting Stock Return Volatility (2021). Swiss Finance Institute Research Paper No. 21-95, https://ssrn.com/abstract=3995529
Further questions
What's your question? Ask it in the discussion forum
Have an answer to the questions below? Post it here or in the forum
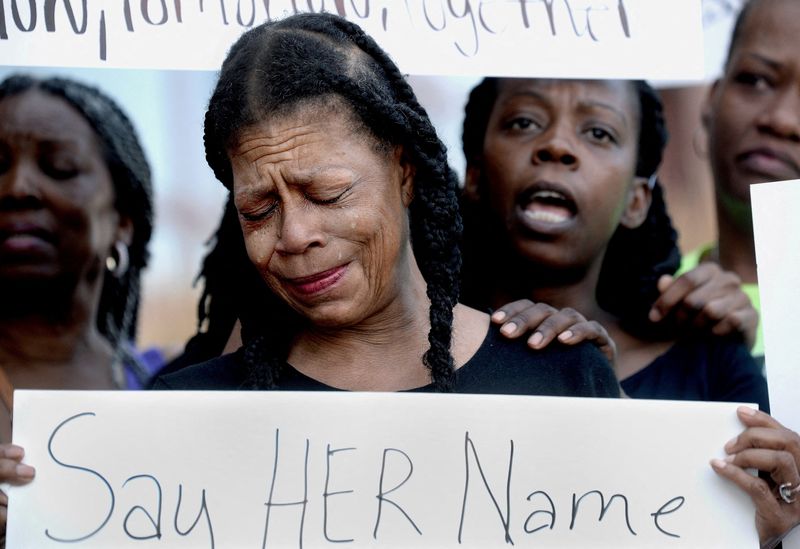
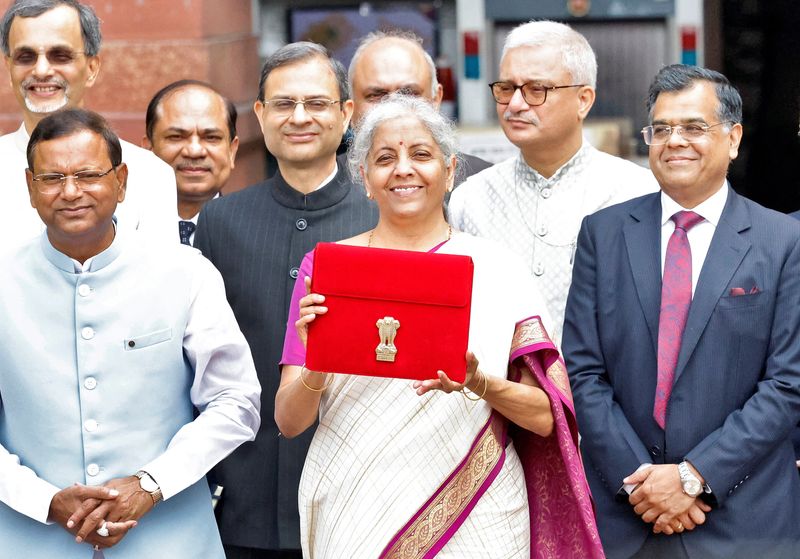
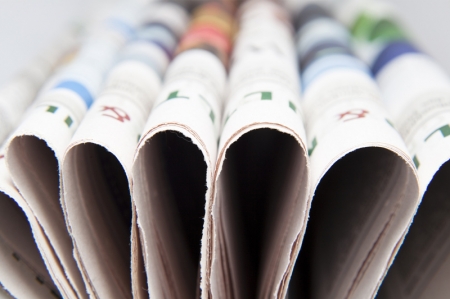

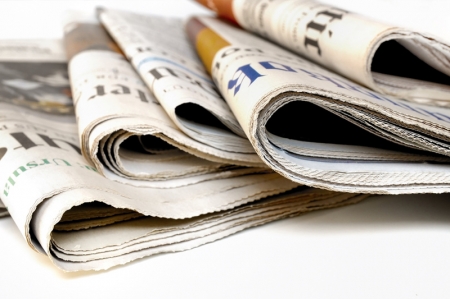