Follow us on LinkedIn
For processes that involve analyzing a high amount of data, sampling is a critical process. However, for it to be effective, the user must select the sample properly. Nonetheless, it might not be possible to do so because some bias may exist when users choose a sample from a given population. There are various types of biases that cause users to select the wrong sample.
What is Survivorship Bias?
Survivorship bias is a type of bias caused during sampling selection. It occurs when investors only consider the surviving or existing observations but fail to take into account data that no longer exists. Practically, survivorship bias happens when investors examine the performance of only existing stocks in the market and regard them as a representative sample while disregarding stocks that have gone bust.
When the process of sample selection gets affected by survivorship bias, it can result in wrong decisions made by investors. For example, by not considering stocks that no longer exist, investors miss out on vital information on what caused those companies to cease. There are multiple factors that investors miss through survivorship bias in their decision-making.
How does Survivorship Bias affect investors?
Survivorship bias makes investors select samples that show a positive view of the market while avoiding its negative aspects. This bias exists because it only considers optimistic samples that have survived due to their steadfastness in strenuous conditions but do not consider those that failed in similar conditions. By not considering the failures, investors make decisions only based on successful histories.
A sample selection based on survivorship bias does not show the whole picture. While the existing data is what investors need to make decisions about, they also need to consider the other failed items in the population to get a representative sample. Furthermore, survivorship bias also causes the results of the analysis performed by investors to skew higher.
How to prevent Survivorship Bias?
Preventing survivorship bias is straightforward. When making a selection of samples, investors need to be aware of its existence. Therefore, they need to not only consider the successful stocks or samples but also look for items that they would not examine. Similarly, investors need to review their data sources to ensure they present both surviving and non-surviving data about their analysis.
Another way to prevent survivorship bias is for investors to not rely too heavily on past returns when making their decisions regarding investments. When investors have a limited view while performing analysis, they are bound to miss vital information during the process. Similarly, they need to ensure they select a representative sample in the selection process.
Example
An investor considering investing in mutual funds comes across the following data.
Mutual Fund no. |
Historical return |
Active/inactive |
1 |
5% |
Active |
2 |
-3% |
Inactive |
3 |
7% |
Active |
4 |
-4% |
Inactive |
If the investor considers the active mutual funds only, their average return will be 6%. However, if they vet the inactive mutual funds in the equation as well, the average return would be 1.25%. Therefore, their decision-making can substantially change by considering those non-existent mutual funds along with active ones. If they don’t evaluate the inactive mutual funds, it results in a sample selection based on survivorship bias.
Conclusion
In investing, survivorship bias occurs when investors fail to consider the data that is no longer active. For example, when investors fail to take into account the stocks that no longer exist when selecting a sample of stocks. Survivorship bias can have negative impacts on the decision-making of investors. However, it is still preventable.
Further questions
What's your question? Ask it in the discussion forum
Have an answer to the questions below? Post it here or in the forum
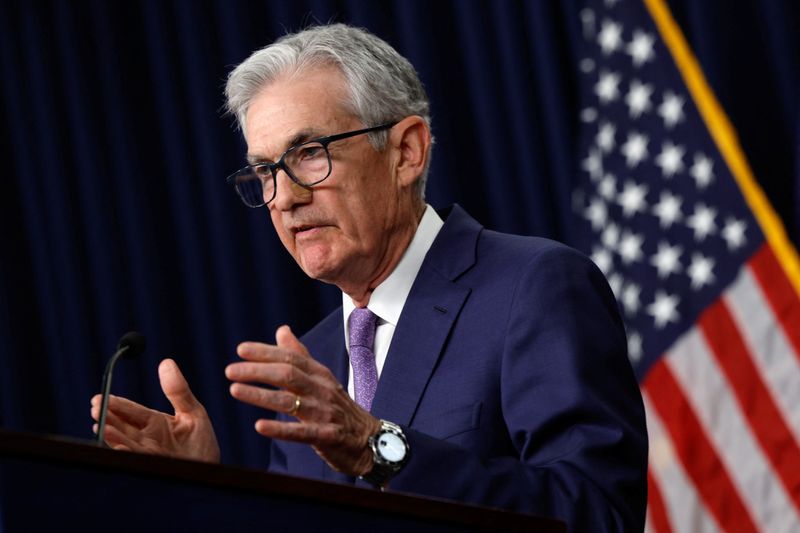
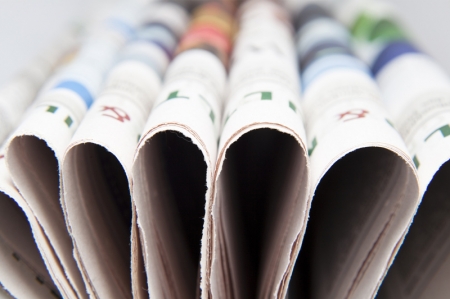
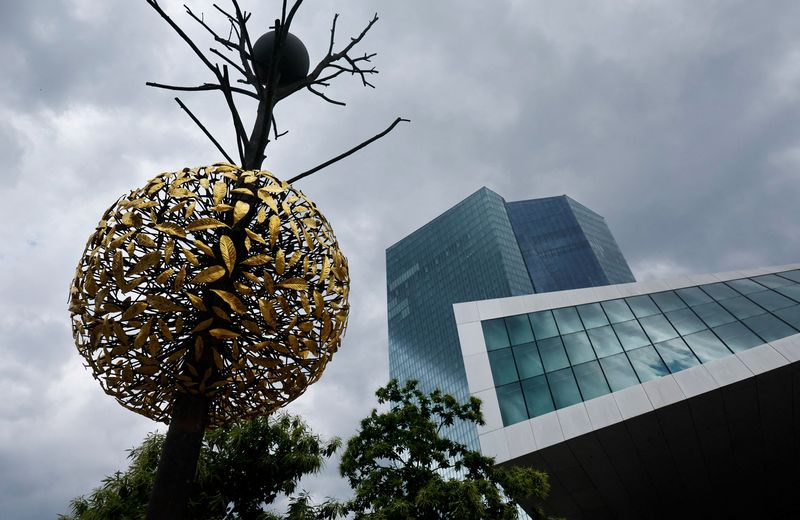
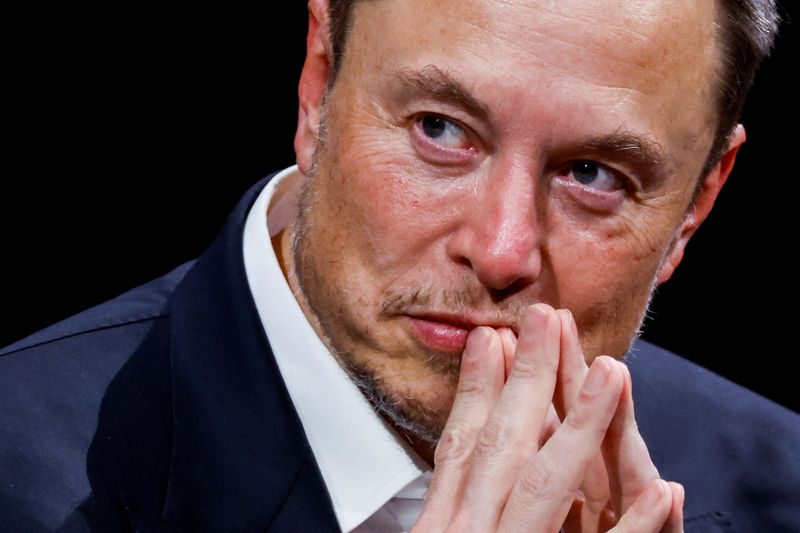

Storms moved into Indiana and Michigan later Monday night, prompting additional alerts including multiple tornado warnings in Indiana.