Machine learning is a subset of artificial intelligence that involves training algorithms to learn patterns from data and make predictions or decisions without being explicitly programmed. It encompasses a range of techniques, from simple linear regression to complex neural networks, and is used in various applications such as image and speech recognition, fraud detection, personalized recommendations, and finance.
In finance, machine learning (ML) has been applied to developing trading strategies. Reference [1] examined the effectiveness of ML in trading system design. Specifically, it investigates how ML-aided trading strategies perform out-of-sample. The authors pointed out,
This paper analyzed the impact of hyperparameter settings on the predictive performance of support vector machines for stock price movement directions. We tested different settings of hyperparameters in an extensive grid search, applying 10-fold cross-validation for the training set and evaluating the classification performance and strategies’ profitability for out-of-sample data. The results indicated that most models had a high in-sample accuracy but only around 50% when applied to out-of-sample data. Nonetheless, a significant proportion of the models managed to outperform the buy-and-hold strategy in terms of profitability. Specifically, several of the models had an out-of-sample F1 Score close to 65%; however, the models that yielded the best profitabilities followed a chaotic behavior, as the out-of-sample performance of the models showed significant oscillations for small variations on the hyperparameters.
In brief, the authors concluded that overfitted trading strategies, which often have nearly 100% in-sample accuracy, typically have only around 50% out-of-sample accuracy, which is in agreement with the Efficient Market Hypothesis.
The authors also found that the most profitable trading strategies are sensitive to variations in parameters. While we agree with the rest of the article, we don’t agree with this point. In our experience, the most robust strategies exhibit little sensitivity to parameter variation.
Let us know what you think in the comments below or in the discussion forum.
References
[1] Yaohao Peng, João Gabriel de Moraes Souza, Chaos, overfitting and equilibrium: To what extent can machine learning beat the financial market?, International Review of Financial Analysis Volume 95, Part B, October 2024, 103474
Further questions
What's your question? Ask it in the discussion forum
Have an answer to the questions below? Post it here or in the forum
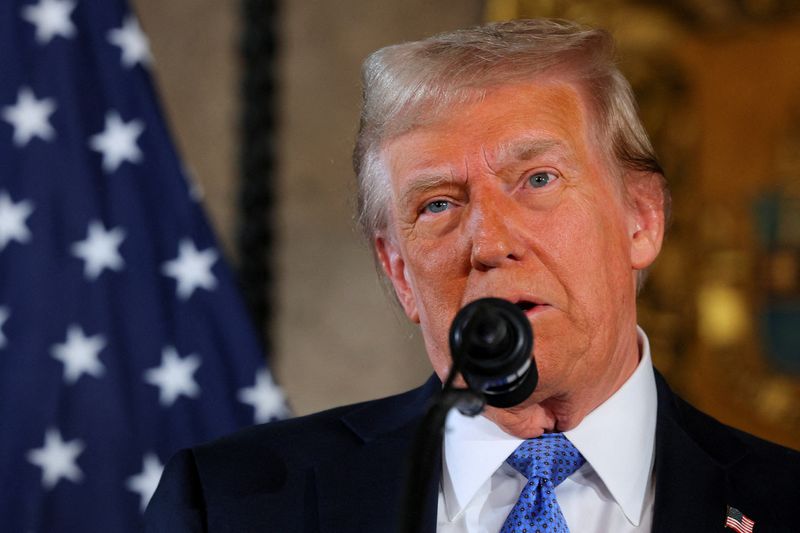
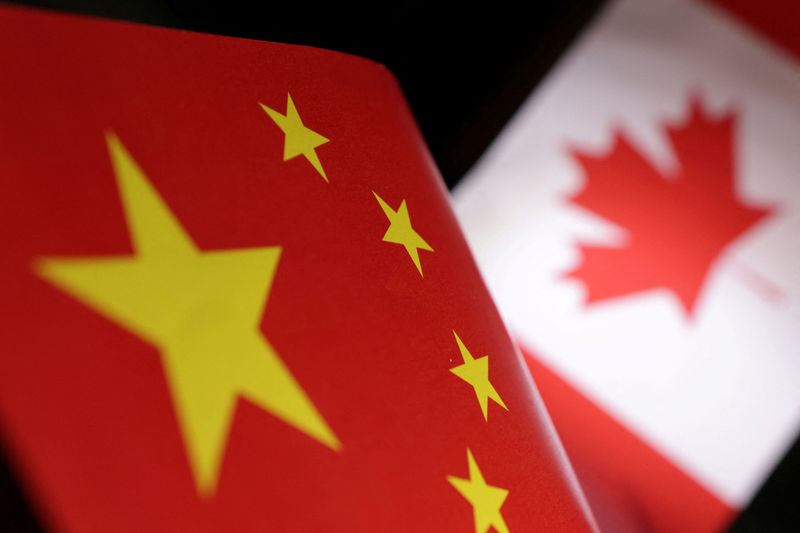
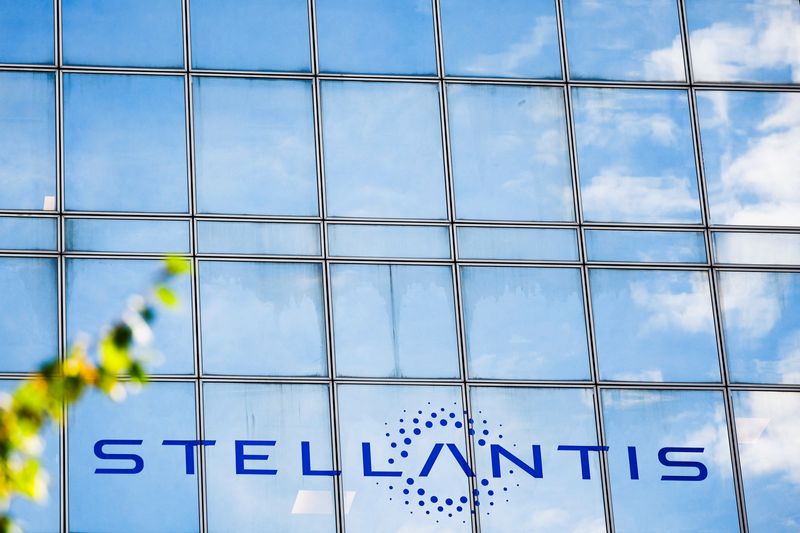
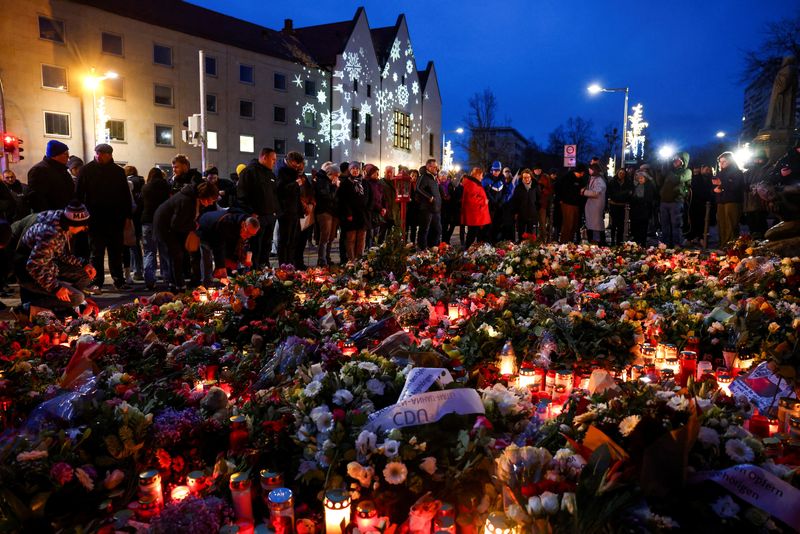
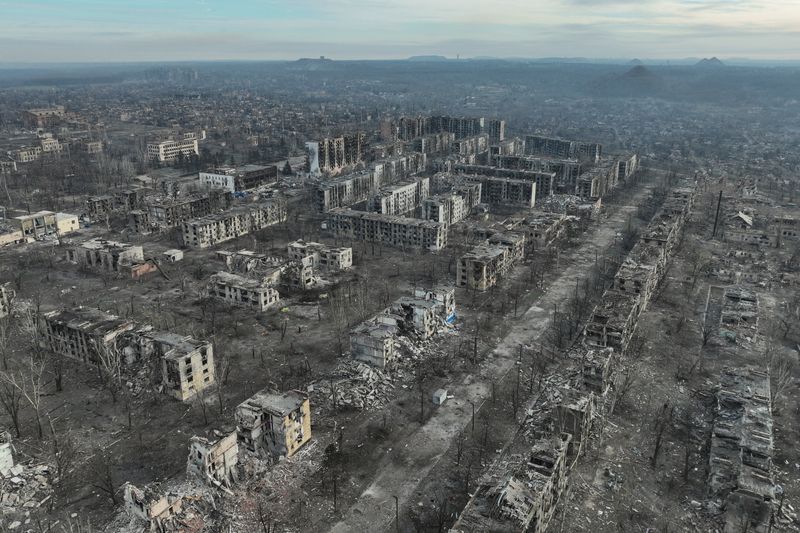