Follow us on LinkedIn
The Central Limit Theorem (CLT) is a concept from statistics, which states that the sample mean distribution of a random variable approaches a normal distribution as the sample size increases. Briefly, it suggests that the sampling distribution of the mean resembles normal distribution with an increase in the size of the sample, regardless of the shape of the original distribution.
For example, a user has a distribution that considers 20 samples. As they increase their size from 20 to 30 or 40, the distribution will approach a normal distribution. The theorem also suggests that a user must consider at least 30 samples for it to be true.
How does the Central Limit Theorem work?
The central limit theorem suggests that the random samples of a random population variable, regardless of its distribution, will approach a normal probability distribution when the sample population exceeds 30. However, as the user increases the number of samples considered, the graph will start resembling a normal distribution more and more.
With the central limit theorem, the average of the sample means and standard deviation will equal the population mean and standard deviation. Therefore, users can utilize it to predict the characteristics of the population.
How can Central Limit Theorem help in finance?
The central limit theorem helps researchers or analysts in predicting the mean and the standard deviation of a population with the help of a sample selected from it. Since it requires a randomly selected sample size of larger than 30, any sample size selected will approach a normal distribution, which further helps in hypothesis testing and constructing a confidence interval for it.
In finance, investors use the central limit theorem when examining the returns of a stock or indices because it helps with easier analysis. Similarly, investors use the theorem when creating their portfolio or managing the risk related to it. The central limit theorem is easy to use as the financial data necessary to construct it is straightforward to obtain.
For example, investors can select any 30 random stocks from an index to predict the population with the help of the sample as it will follow a normal distribution. Similarly, the mean and the standard deviation of the selected sample of stocks will be the same as the mean and the standard deviation of the overall index. Therefore, it can help them with decision-making about investing in the index as a whole.
What are the characteristics of the Central Limit Theorem?
The central limit theorem exhibits a few characteristics. Firstly, the mean of the sample means is equal to the mean of the population from which investors select the sample. Similarly, as mentioned above, the sampling distribution to approximate a normal distribution should have a sample size larger than 30. Lastly, the standard deviation of the distribution of sample means will equal the population standard deviation divided by the square root of the sample size (σ/Sqrt(n)).
Conclusion
The central limit theorem is a topic of importance in statistics. It suggests that the sample mean distribution of a random variable will approach a normal distribution with an increase in the sample size. However, the sample size should be equal to or exceed 30 to achieve a normal distribution.
Further questions
What's your question? Ask it in the discussion forum
Have an answer to the questions below? Post it here or in the forum
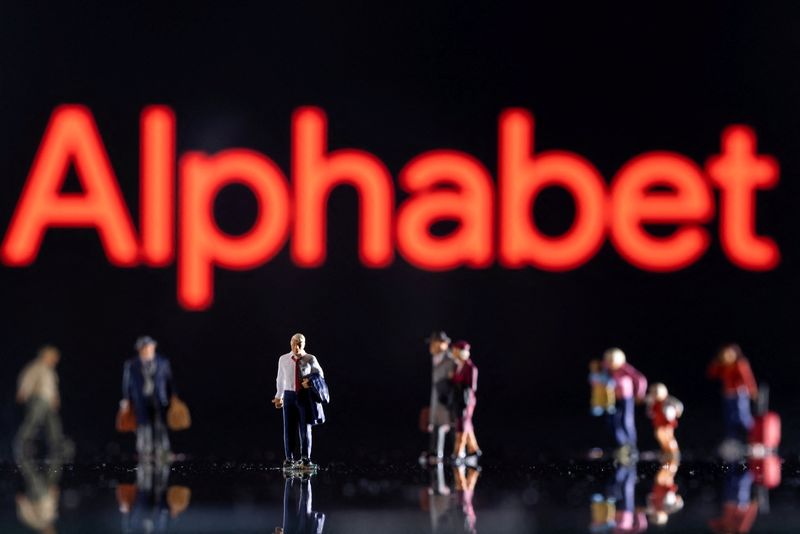
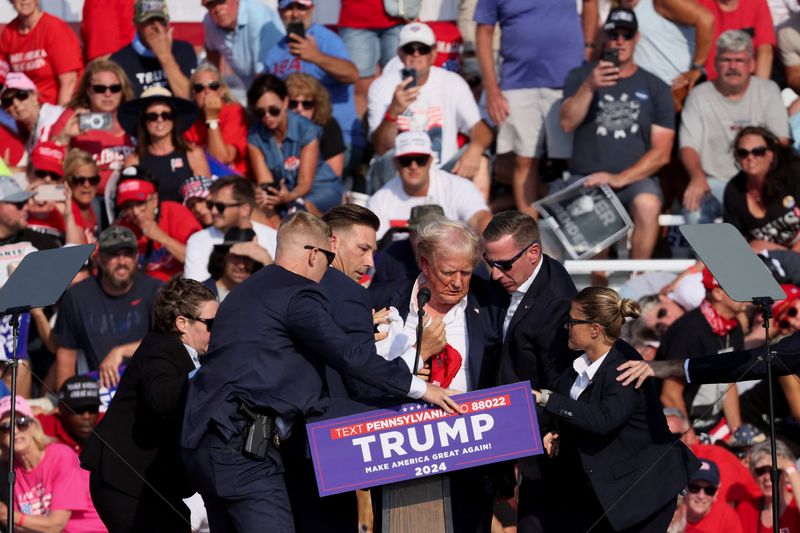
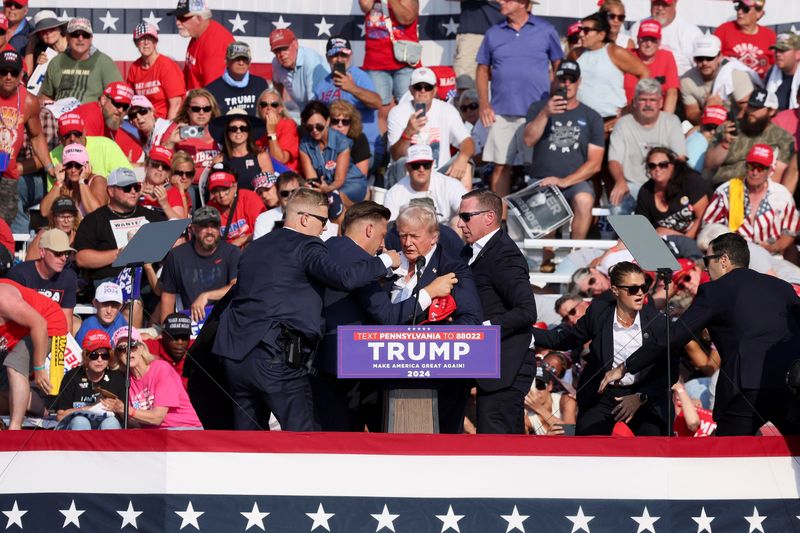
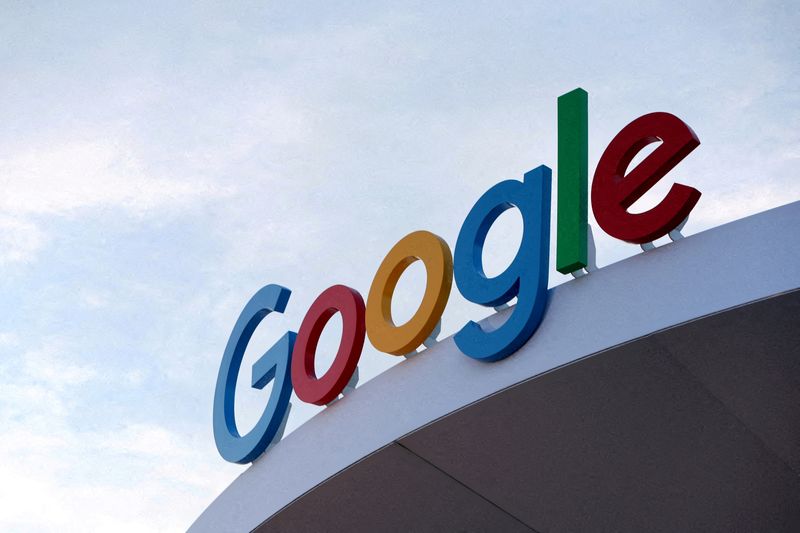
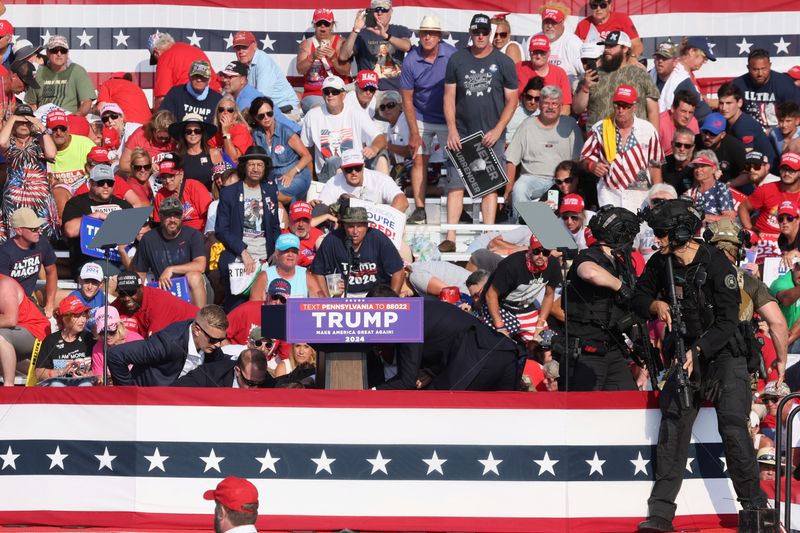