Geometric Brownian Motion (GBM) is a widely used mathematical model for simulating the random behaviour of asset prices in financial markets. It assumes that the price of an asset follows a continuous-time stochastic process, where the logarithmic returns are normally distributed. GBM is foundational in option pricing models like Black-Scholes-Merton.
Despite its widespread use, the GBM model has limitations. Reference [1] addressed these limitations by incorporating long memory (long-range dependence) and stochastic volatility into the GBM framework. Three models were studied,
- Model 1, the classic GBM, which excludes both memory and stochastic volatility,
- Model 2, the fractional geometric Brownian motion (FGBM), which includes memory but ignores stochastic volatility, and
- Model 3 incorporates both memory and stochastic volatility.
The study empirically analyzed these models by forecasting the Euro exchange rate against three currencies: Saudi Riyal (SAR), US Dollar (USD), and Australian Dollar (AUD). The authors pointed out,
Exchange rates play a crucial role in the financial trade of any country, especially in international trade. Therefore, understanding the future direction of exchange rates is a priority for stakeholders. To achieve this goal, many researchers in the literature have proposed several models. In this study, the researchers utilized three GBM-based models to predict the exchange rates of three currency pairs: EUR/USD, EUR/SAR, and EUR/AUD. The first model followed the traditional GBM approach without considering memory or assuming stochastic volatility. The second model, known as GFBM, incorporated memory but ignored the assumption of stochastic volatility. Finally, the third model, also a type of GFBM, took both memory and stochastic volatility into account. After performing predictions with all three models, it was observed that the third model demonstrated superior performance, as evidenced by its lowest Mean Squared Error (MSE). This result indicates that incorporating memory and assuming stochastic volatility in GBM positively impacts its effectiveness as a tool for predicting exchange rate prices. Therefore, given the high accuracy shown by model 3, it can confidently be used for forecasting future exchange rates.
In short, the findings suggest that incorporating long-range memory and stochastic volatility significantly enhances the model’s predictive power.
Let us know what you think in the comments below or in the discussion forum.
References
[1] Mahan Farzina, Mehdi Sadeghi Moghaddamb, Amir Mohammad Shahbalaei Kashan, The Effects of Adding Memory and stochastic volatility in the GBM Method for Predicting the Euro Exchange Rate, Applied Innovations in Industrial Management 4-1 (2024) 30–41
Further questions
What's your question? Ask it in the discussion forum
Have an answer to the questions below? Post it here or in the forum
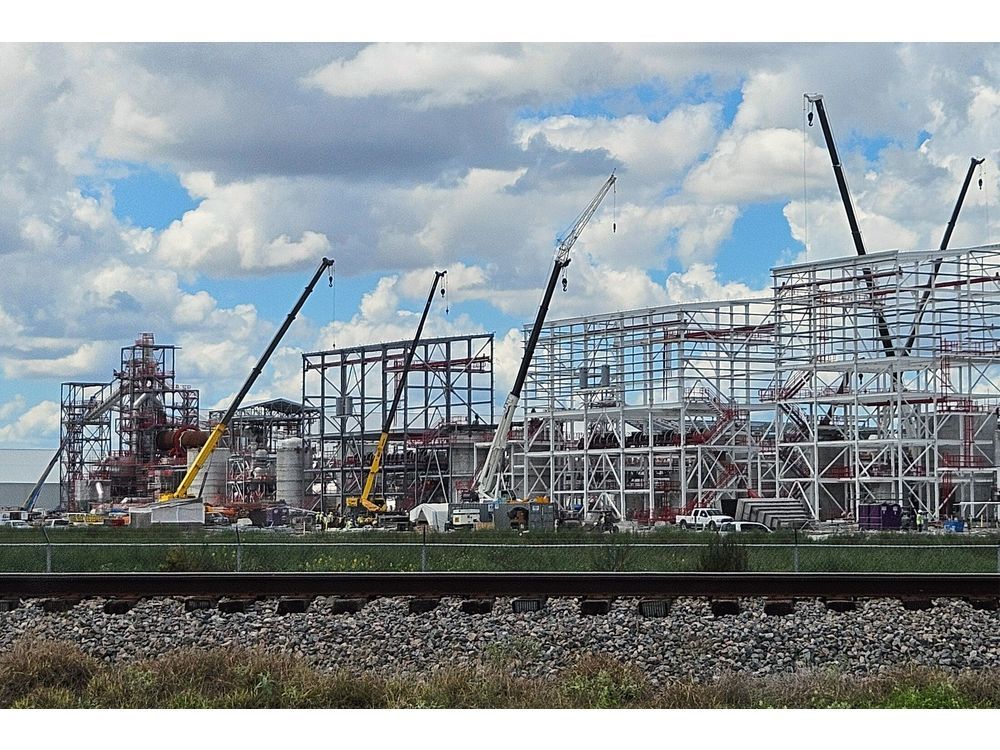
A lithium factory in a dry Gulf Coast county epitomizes the EV maker’s build now, ask later ethos.
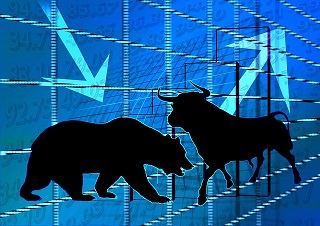
Acquired a fledgling company less than 18 months old for 100 times its initial capital. Acquisition negotiations began just five months after Igneo’s establishment —a highly unusual move. Major shareholder MCC NFT and investment funds like PCT reportedly realized extraordinary returns. SEOUL, South Korea —…
Laid off and applying for a new job? An HR exec with 36 years of experience shares scripts for discussing being made redundant in an interview.
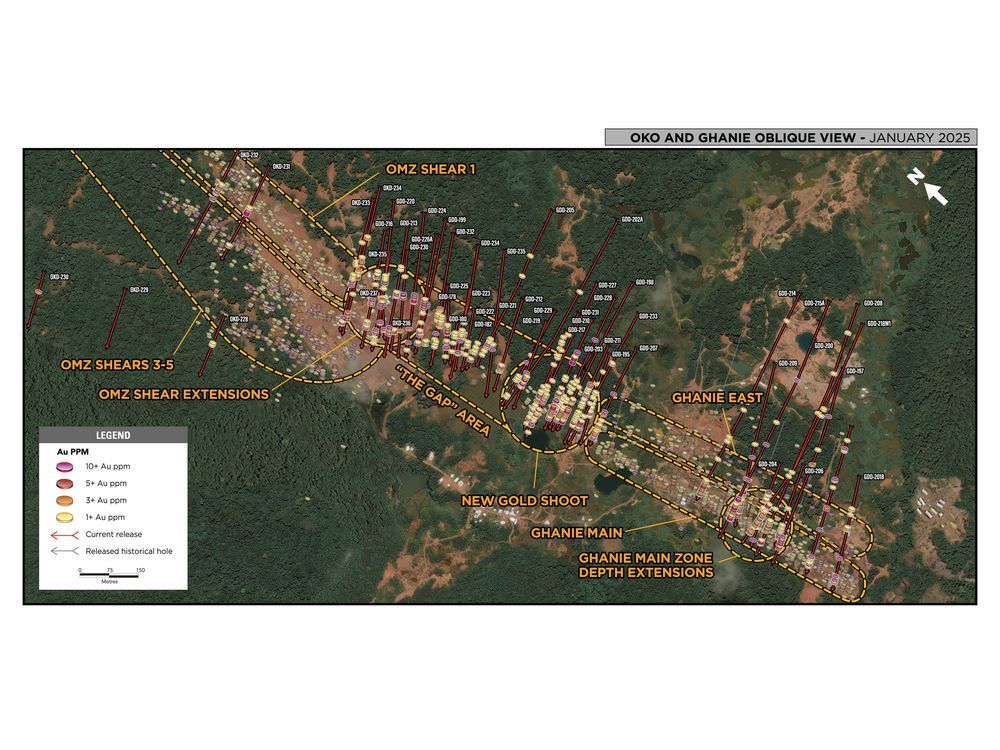
New high grade shoot discovered between Ghanie and Oko gold zones – 13.5m @ 5.7 g/t Au & 4m @ 36.8 g/t Au Ghanie Main Zone extended to depth with high grade intercepts – 4m @ 11.6 g/t Au & 3m @ 12.1 g/t Au…
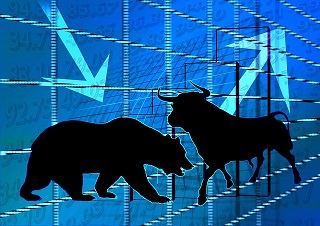
BANGKOK (AP) — U.S. President Joe Biden’s decision to reject a bid by Nippon Steel to acquire U.S. Steel on national security grounds isn’t the first time friction over trade and investment has irked Washington’s closest ally in Asia. There have been plenty of scraps…