Follow us on LinkedIn
Volatility forecasting plays an important role in finance. Its importance lies in the fact that it enables us to effectively price options and plan trading and hedging activities. By providing insights into future market volatility, this forecasting becomes an essential tool for investors, enabling them to make informed decisions that align with their risk tolerance and financial goals.
Many volatility forecasting methods rely on underlying asset prices, but in Reference [1], the authors introduced a technique that incorporates trading volume. They employed an approach known as the heterogeneous autoregressive model for realized volatility (HAR-RV), and integrated average trading volumes from various timeframes, including one, five, and 22 trading days. The authors pointed out,
Within the framework of HAR-RV type models we have investigated whether utilizing, in the spirit of HAR modeling, past average trading volume for long periods – week and/or month – are able to improve the quality of volatility forecasting. We compare models with past average volume for a day, week and month and models with past average volume for a day and week, on the one hand, and the model without volume and the model with past daily trading volume only. We employ an out-of-sample R2 and the model confidence set procedure driven by MSE or QLIKE loss functions. We find that typically, for the one-day forecasting horizon, including past average week volume or average week and month volumes improves, statistically and economically significantly, and never worsens, forecasting quality relative to the benchmark models. The improvements are weaker at the one-week forecasting horizon, and rarely, if ever, appear at the one-month horizon.
In short, including volume data enhances one-day volatility forecast accuracy, but its usefulness diminishes when predicting volatilities over one week or one month.
The authors also provided an explanation for the importance of trading volume,
We have suggested two explanations of these results, one based on different flows of information coming to the market in periods of different length, and the other based on the division of labor among different-length-periods average volumes in containing different types of information – shocks coming to the market and how fast information spreads out in the market.
Let us know what you think in the comments below or in the discussion forum.
References
[1] Stanislav Anatolyev, Sergei Ponomarev, Does trading volume help forecast volatility?, 2023, https://pages.nes.ru/sanatoly/Papers/VolVol.pdf
Further questions
What's your question? Ask it in the discussion forum
Have an answer to the questions below? Post it here or in the forum
The Princess of Wales will present the trophy to either last year's winner, Carlos Alcaraz, or seven-time Wimbledon champion, Novak Djokovic.
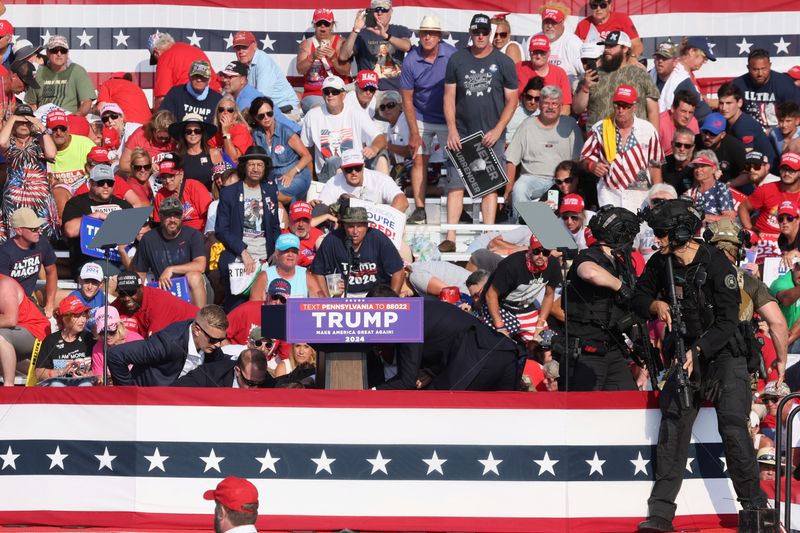
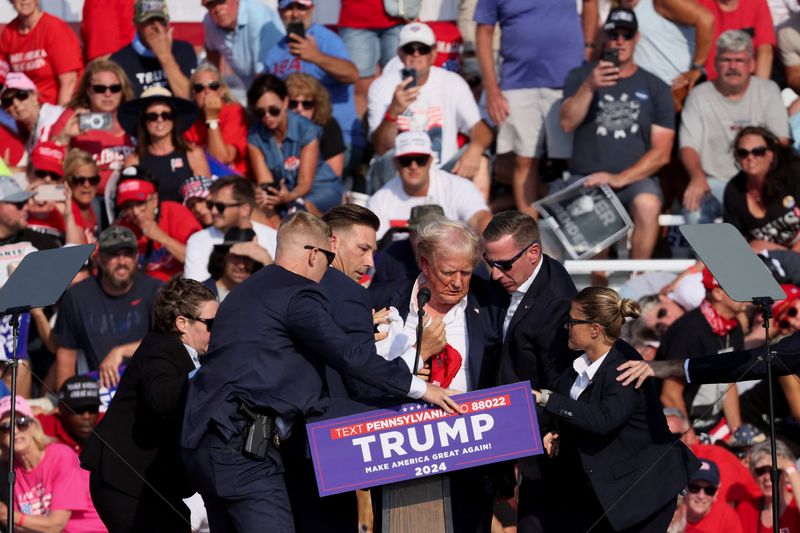
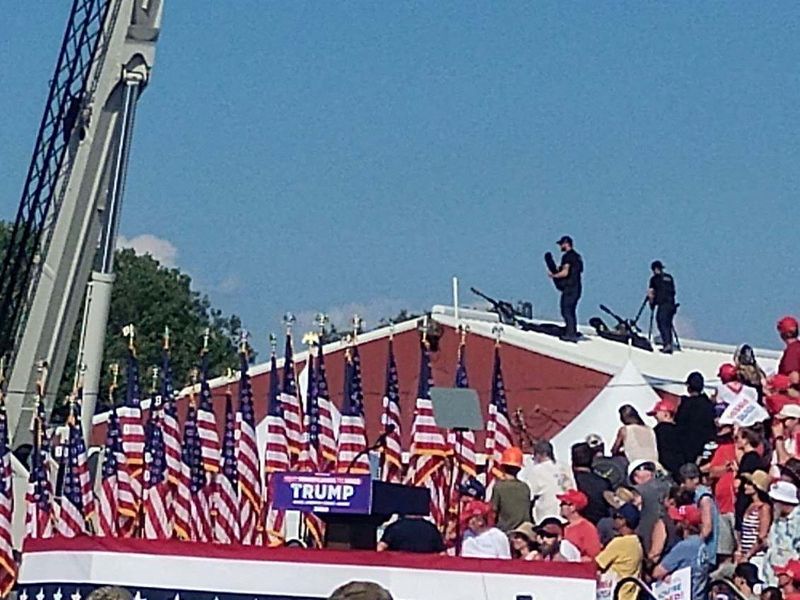
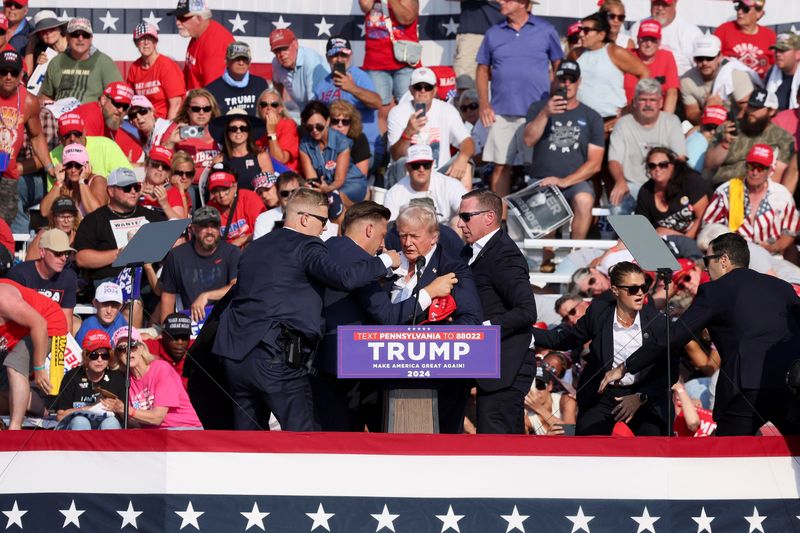