Follow us on LinkedIn
Statistical arbitrage is a trading strategy that exploits the differences in prices between different asset classes. It has been employed, however, not only in the financial market but also in sports betting.
Sports betting is a form of gambling that involves wagering on the outcome of a sporting event. Of all the sports, football (soccer) is the most popular one for betting. The basic idea behind sports betting is to forecast the outcome of a match, and then place a bet accordingly.
There are different types of bets that can be placed on a football match. The most common bet is the 1X2 bet, which predicts the winner of the match. Another popular bet is the Goal Line bet, which predicts the number of goals that will be scored in the match. There are also other bets, such as the halftime/fulltime bet, the first goal scorer bet, and so on.
The most common approach used for building a predictive model in football betting is based on some sort of ranking system. Every team is assigned a certain number of points, and the team with more points is expected to win. The ranking can be based on different factors, such as the number of goals scored, the number of matches won, and so on.
Reference [1] proposed a novel approach that utilizes not only the teams’ rankings but also players’ characteristics,
Our work delivers a machine learning framework for forecasting future football matches and achieving excess returns through appropriate betting. To be more specific, the profits are generated by exploiting a large amount of data about characteristics of both the match and the players involved. In the simulation study, our approach combining different machine learning algorithms achieved economically and statistically significant returns of 1.58% per match. Thus, our results confirm the statement of the existing literature that forecasting football league match results pays off.
In short, statistical arbitrage is profitable in the football market. The authors also outlined the direction for future research,
In the short-term future, the presented statistical arbitrage framework could be used to forecast the results of other sporting events, e.g., rugby, American football, or basketball. Machine learning is also relevant in the area of individual sports, such as tennis or golf, since individual player skills play a very important role. Finally, the information about the time of the data could be an important feature to increase the accuracy of our model.
Given that the developed method takes into account players’ characteristics, we believe that it can be applied in other areas of the football market, for example, to price players’ contracts, and develop strategies for winning a match or tournament.
References
[1] Johannes Stübinger, Benedikt Mangold, and Julian Knoll, Machine Learning in Football Betting: Prediction of Match Results Based on Player Characteristics, Appl. Sci. 2020, 10, 46
Further questions
What's your question? Ask it in the discussion forum
Have an answer to the questions below? Post it here or in the forum
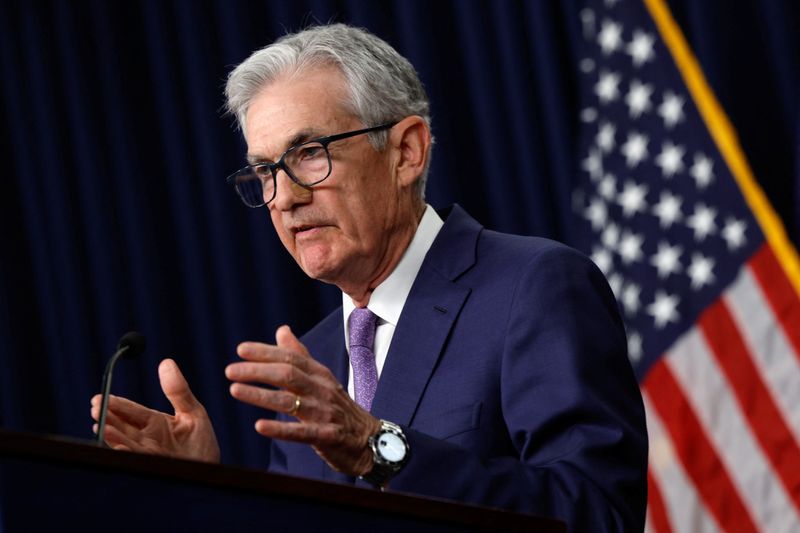
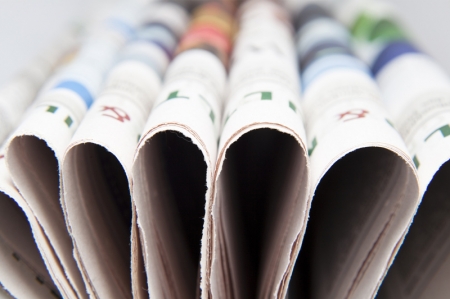
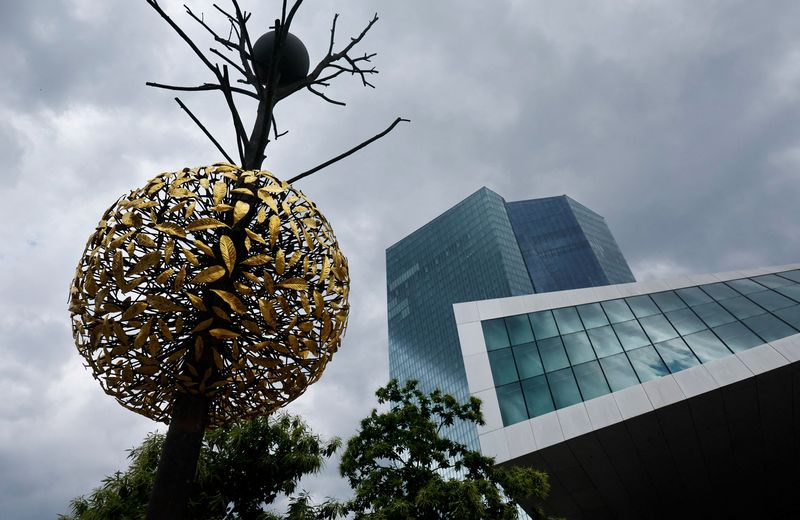
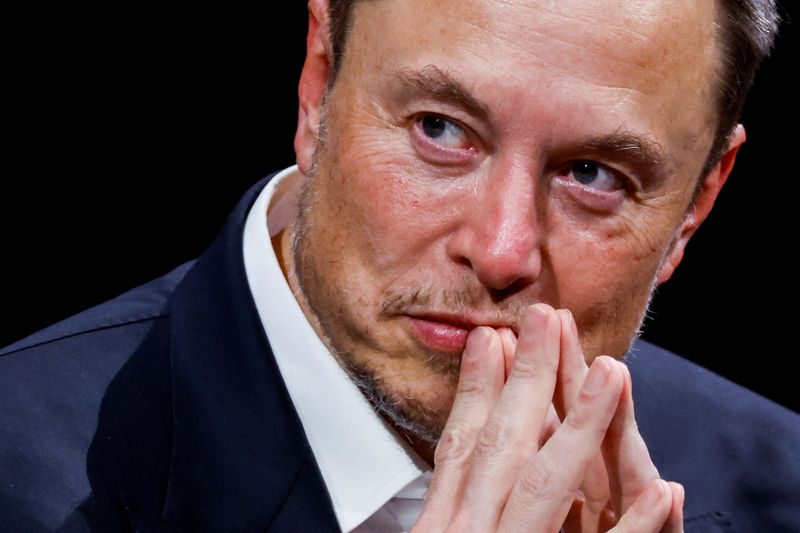

Storms moved into Indiana and Michigan later Monday night, prompting additional alerts including multiple tornado warnings in Indiana.