Follow us on LinkedIn
Bitcoin was born as a niche investment vehicle, but over time it has become a mainstream asset. Many research papers have been devoted to developing trading strategies for Bitcoin, but we just recently came across one [1] that examined the PnL/risk driver in terms of autocorrelations and developed trading systems accordingly.
Specifically, the paper investigated the autocorrelation properties of Bitcoin in different timeframes. It pointed out that, like stock indices, Bitcoin is mean-reverting. But unlike stock indices, Bitcoin is mean-reverting across a broader spectrum of timeframes.
We analyzed the intraday behavior of Bitcoin, comparing the features of its market with the stylized facts that characterize the series of traditional financial assets such as stocks and foreign exchange pairs. The comparison was based on data recorded between March 2015 and June 2018, and highlighted several similarities as well as some important differences. Among those differences, the primary finding was the presence of significant negative first-order autocorrelation in the series of Bitcoin returns, not only limited to extremely high-frequency returns, but extended to medium-frequency ones, such as those computed at intervals of one, two and four hours.
The author subsequently provided main reasons for the mean-reverting nature of Bitcoin, namely (i) investor and trader overreaction, (ii) excess volatility, and (iii) cascading liquidations due to excessive use of leverage by market participants.
Significant negative autocorrelation of returns indicates that price tends to systematically mean-revert in the period immediately subsequent to a move. We attribute this finding to a combination of factors. Among them, we believe the most important ones to be investor and trader overreaction, high volatility, and excessive use of leverage leading to liquidation cascades.
Trading strategies were developed based on research results, and they’re profitable across a broad range of timeframes and triggers,
…a strategy that checks price every five minutes, then goes long if the return in the last five minutes was negative and greater in absolute value than two standard deviations, or goes short if the return in the last five minutes was positive and greater than two standard deviations. Each trade is then closed after a single period, regardless of the outcome. In our example, this means that each trade only lasts five minutes. The results are significantly positive across the board, meaning that even with a basic strategy like the one used, the negative autocorrelation of returns would likely be exploitable, assuming market microstructure to be similar to that of legacy markets.
We find the research results interesting and promising. However, there are a couple of points worth mentioning:
- The trading strategies were developed with a benefit of hindsight, i.e. the backtest results suffer from the lookback bias.
- Since the negative autocorrelation is the main PnL driver of the trading strategies, going forward it would be crucial to develop an indicator to monitor and signal a regime change, from negatively autocorrelated to random walk or positively autocorrelated.
Finally, we also find the results regarding Bitcoin volatility interesting and relevant.
References
[1] GD Nicola, On the Intraday Behavior of Bitcoin, Ledger, Vol 6 (2021) 58-80
Further questions
What's your question? Ask it in the discussion forum
Have an answer to the questions below? Post it here or in the forum
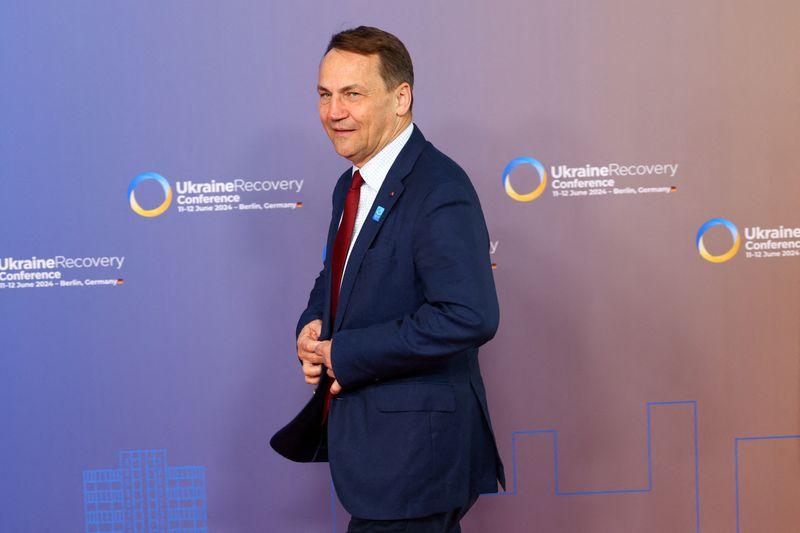
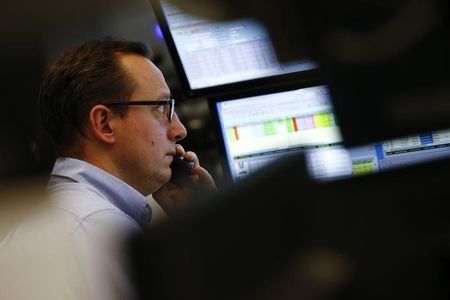
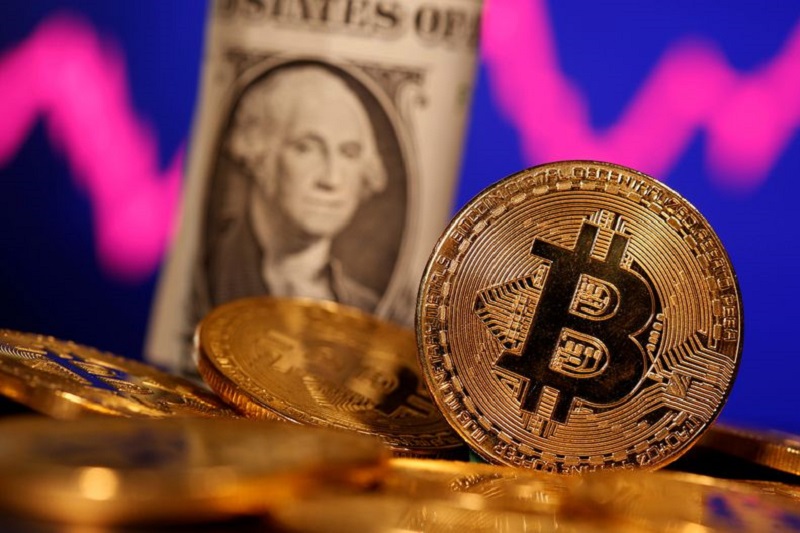
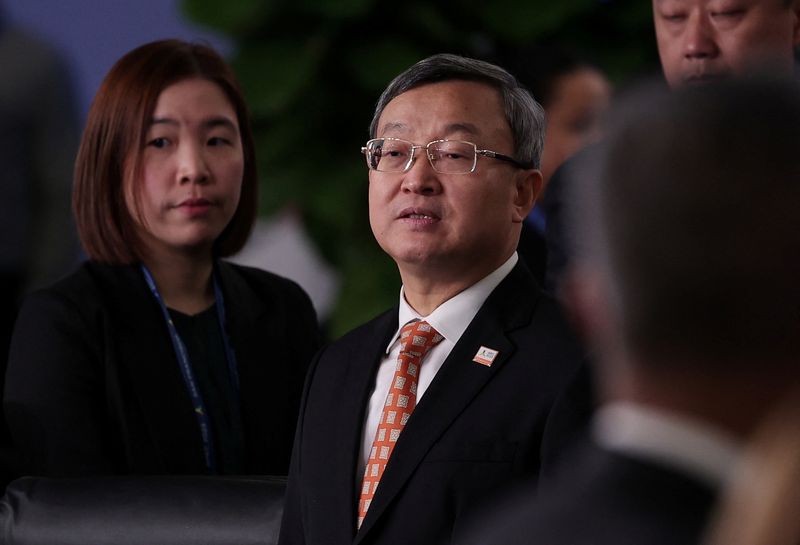
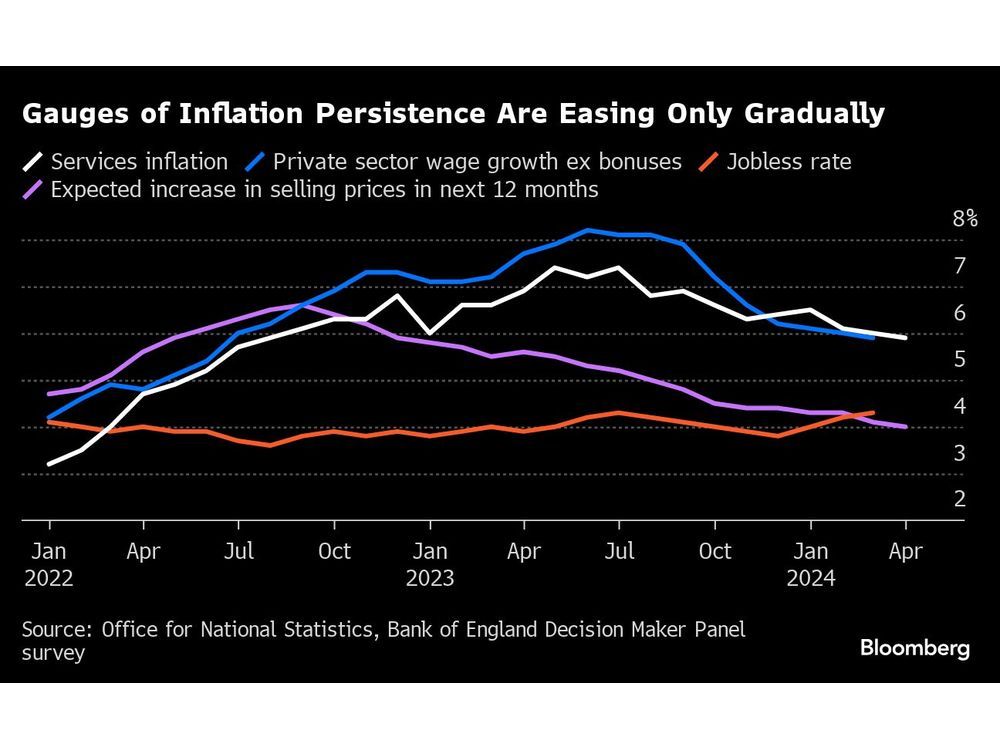
Prime Minister Keir Starmer’s promise to “get Britain building again” will quickly face a shortage of skilled workers in the very industries he’s hoping will power the turnaround.